Pipeline Templates
deepset Cloud comes with a number of ready-made templates you can use out of the box to create a working system like RAG, chat, or document search. Learn what systems you can create out of the box and how they work.
You can view all the available pipelines when you click Pipeline Templates in the navigation. They're grouped by task. You can check what each template does, its applications, output, and the nodes it includes if you view its details.
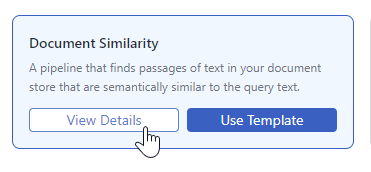
Document Search Pipelines
These pipelines search the document store for files similar to the query text. They can find matches based on keywords, the semantic relationship between the documents and the query, or a combination of both (hybrid search). There are also date-based templates that search for documents, prioritizing the most recent ones.
Document search pipelines use a Retriever or a combination of retrievers and one or more Rankers:
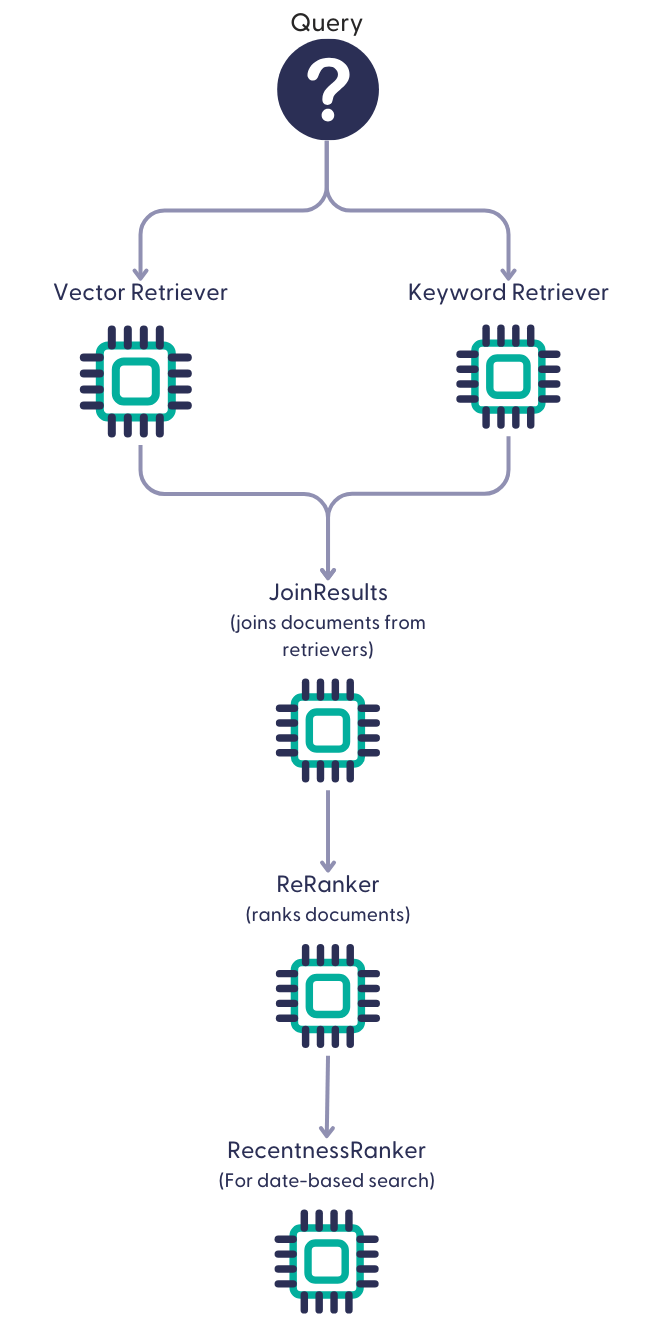
Document search pipelines are the first step of RAG pipelines. The LLM then uses the retrieved documents to generate answers based on them.
You can read more about document search in the Learn section: Document Search.
To enhance your document search pipelines, see Improving Your Document Search Pipeline and Boosting Retrieval with OpenSearch Queries.
Question Answering (QA) Pipelines
Question answering pipelines find answers by extracting them directly from the documents (extractive QA) or by generating new text based on the documents (RAG). You can find basic QA templates, which are extractive and RAG pipelines using different LLMs, and advanced QA templates that include special functions, such as detecting prompt injections or spell-checking.
QA pipelines start with the document search part that fetches documents from the document store and feeds them to the Reader or a PromptNode (answer generator).
Here's how an extractive pipeline may look like:
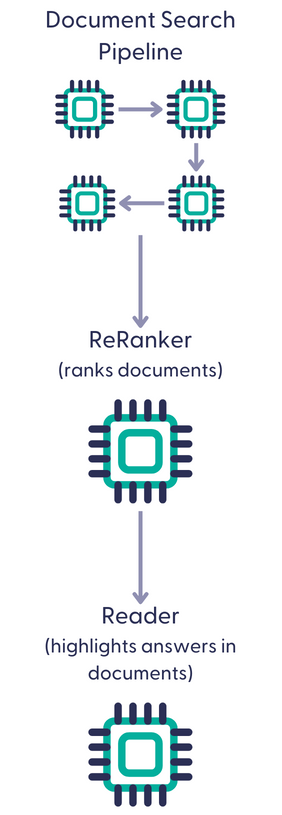
And here's a RAG pipeline:
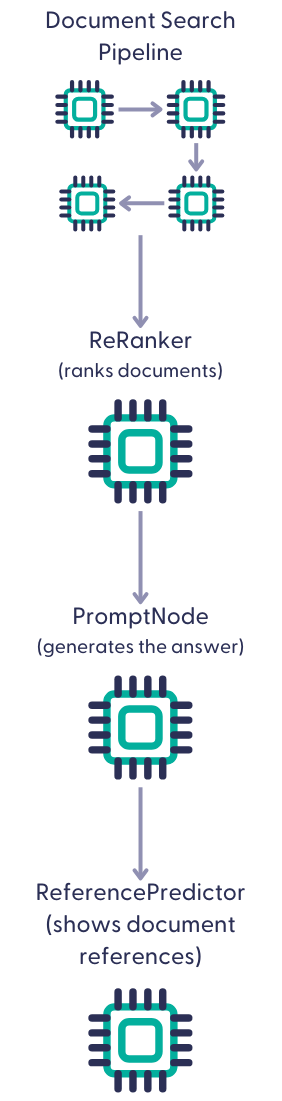
ReferencePredictor adds a reference to each sentence in the generated answer so that you can easily check the answers are grounded in your documents.
More advanced RAG pipelines include additional components that spell-check the question or prevent prompt injection.
To learn more about QA, see Extractive Question Answering and Retrieval Augmented Generation (RAG) Question Answering.
Visual QA Pipelines
With these pipelines, you can query images within your PDF files. Simply upload PDFs to deepset Cloud and then ask questions about them, like you normally would. Visual pipelines look for answers in images and return textual answers.
The document search step uses text available in the PDFs to retrieve relevant documents. Next, a FileDownloader node (called ImageDownloader) converts the parts of the original PDF from which the retrieved documents were created into JPEGs and sends them to the LLM to process. The LLM returns textual answers.
Here's what a visual QA pipeline looks like:
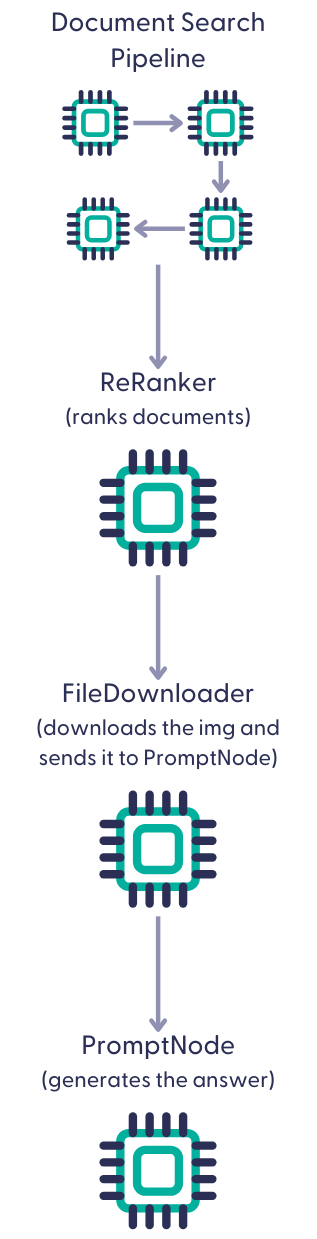
Conversational (Chat) Pipelines
Chat pipelines go beyond traditional search methods by offering a more human-like interaction. Unlike standard search systems that process each query in isolation, chat pipelines remember previous exchanges and provide contextually relevant responses.
In deepset Cloud, chat pipelines run directly on your files. They contain two instances of PromptNode: SummaryPromptNode and QAPromptNode. The SummaryPromptNode retrieves the chat history and refines the user's question to reflect the chat context. It then forwards this contextualized question to the QAPromptNode, which generates an answer.
Whenever a user asks a question, the SummaryPromptNode rephrases it to ensure the QAPromptNode understands it in the broader context of the conversation. For example, if you ask, "What is the capital of Italy?" and then you follow up with, "What should I see there?" the SummaryPromptNode rephrases the question to "What should I see in Rome?". The QA PromptNode is instructed to generate the answer based on the documents it receives in the prompt.
Here's a diagram illustrating a basic chat pipeline:
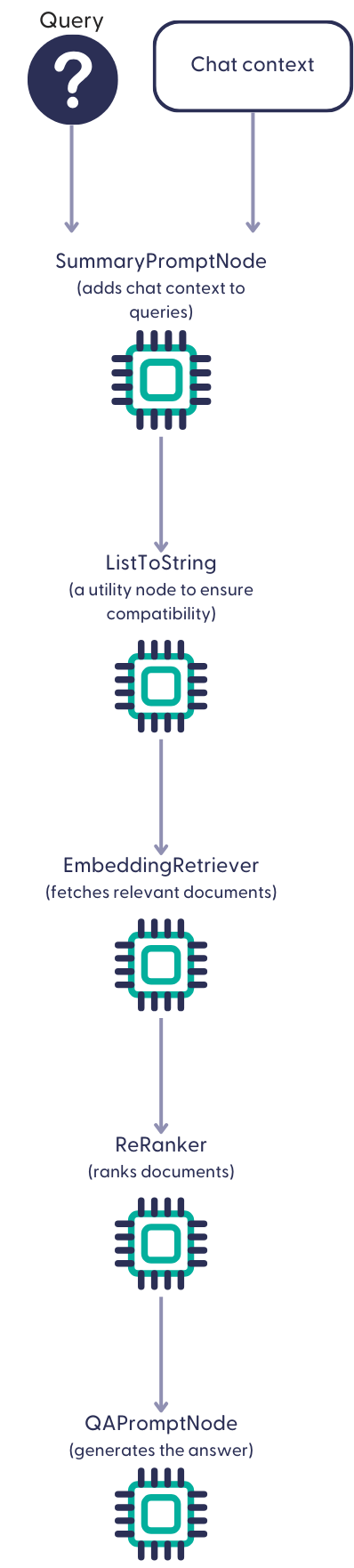
Text Analysis Pipelines
There are three kinds of text analysis pipelines: document similarity, file similarity, and named entity recognition. Document similarity pipelines search the document store for passages of text that are similar in meaning to the query text. File similarity pipelines find files semantically similar to those submitted in the query. Both types use a single retriever to locate and fetch relevant documents from the document store.
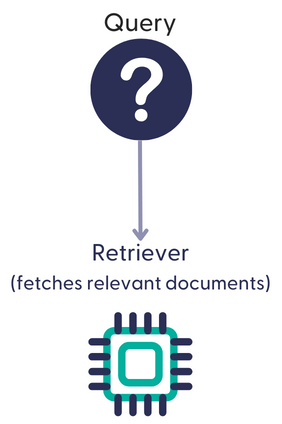
The named entity recognition pipeline identifies persons, organizations, locations, and miscellaneous entities by default. It's an indexing pipeline with a NERNode that runs through preprocessed documents and adds the extracted entities to the documents' metadata. It then sends the enriched documents to the document store.
Text-to-SQL Pipelines
These pipelines are specifically designed to work with databases and are currently optimized for Snowflake. You can use them to interact with your databases using natural language queries. When you type a text query, the pipeline processes it, converts it into SQL and returns both the answer and the SQL table retrieved from the database. This way, you can quickly access your data without writing complex queries yourself.
There are two types of text-to-SQL templates:
- Simple question answering pipeline that accepts text queries and returns an answer together with the SQL table. Use this template if you have up to 50 tables in your database.
- RAG question answering pipeline with an additional retrieval and ranking step before generating the SQL. To use it effectively, you must first prepare table descriptions and upload them to deepset Cloud.
Use this template if you have a large database with over 50 tables.
This diagram illustrates the basic text-to-SQL pipeline:
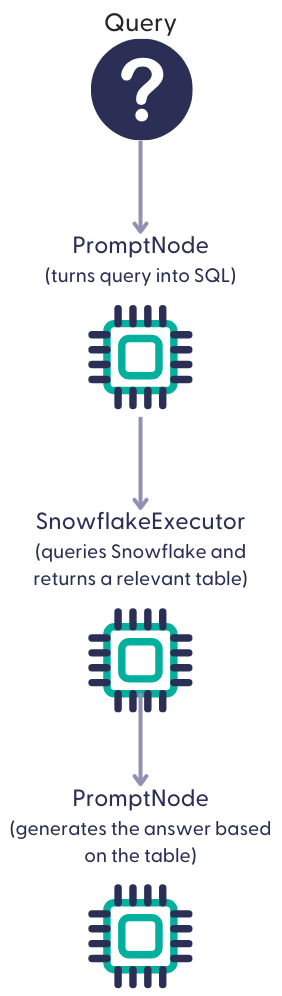
In the RAG text-to-SQL pipeline, the Retriever first fetches the relevant table names based on their descriptions in deepset Cloud and passes them to PromptNode. PromptNode builds a SQL query with these table names and sends it to SnowflakeExecutor, which queries the Snowflake database. SnowflakeExecutor retrieves the relevant table and sends it to PromptNode, which uses it to generate an answer and a resulting table.
Here's what the RAG text-to-SQL pipeline looks like:
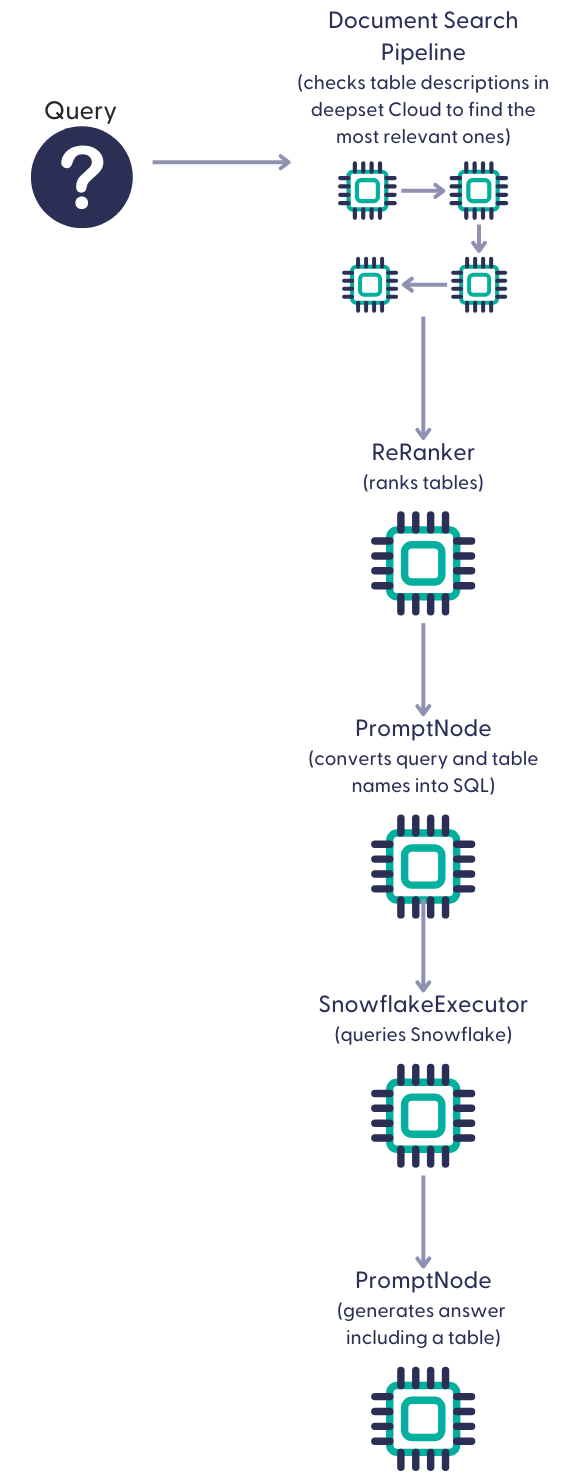
Updated 3 months ago